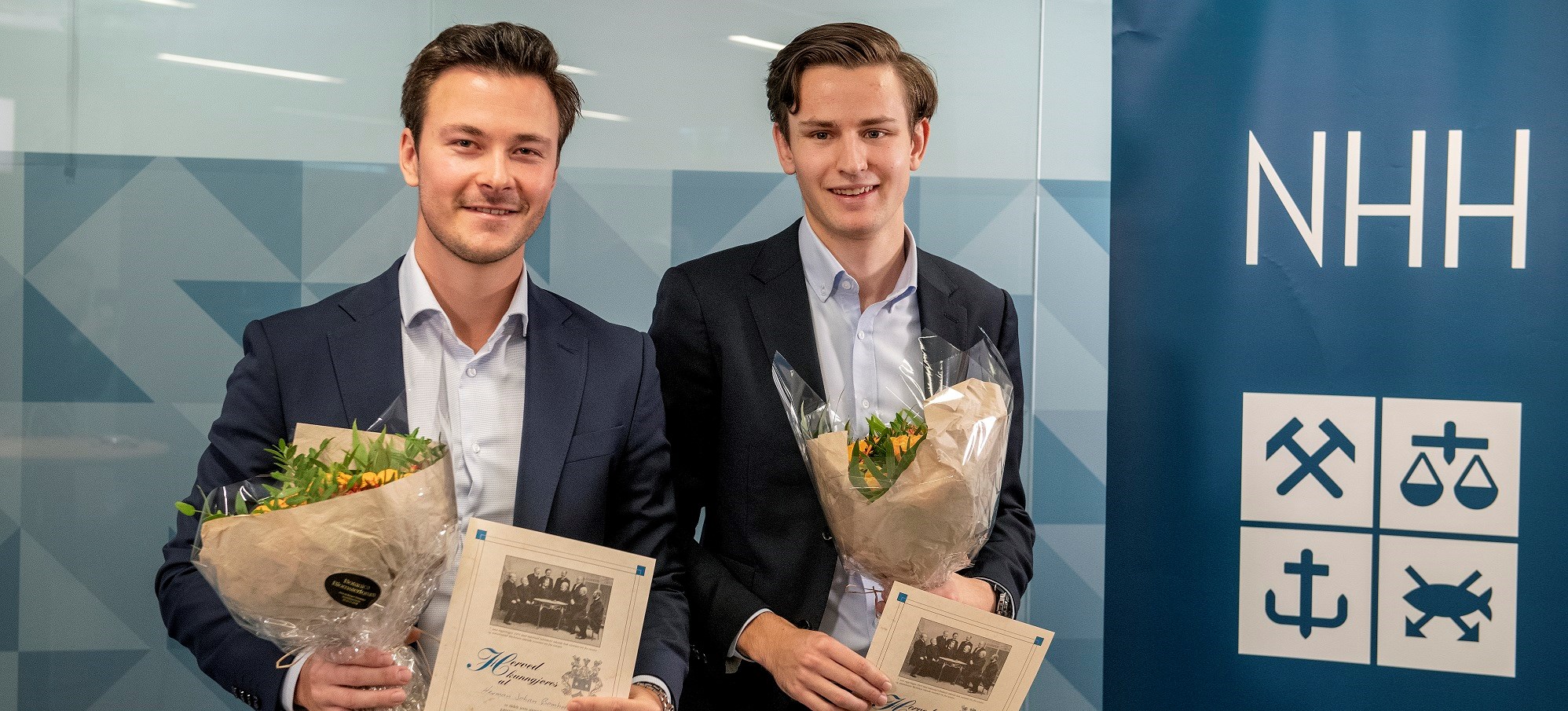
Lehmkuhl grant to master’s thesis about machine learning
Herman Johan Bomholt and Torsten Stangeland Thune were awarded the Lehmkuhl grant for their master’s thesis today.
In connection with the Lehmkuhl lecture this week, Herman Johan Bomholt and Torsten Stangeland Thune were awarded a grant of NOK 25,000 for their master’s thesis Machine learning as a decision support system in Capesize route optimization ─ Predicting optimal route selection using Recurrent Neural Networks and Extreme Gradient Boosting.
Bomholt and Thune submitted their thesis in spring 2020. They were supervised by professor at the Department of Business and Management Science Roar Os Ådland.
The grounds for the decision were outlined as follows:
They demonstrate good insight, both about previous research and practical matters in the industry, and show that they master the use of advanced modelling tools. The system presented is innovative and promising, and the scope of the shipping industry means that the idea may have significant economic potential.
The Lehmkuhl grant is an extra acknowledgement of NHH students or newly graduated candidates who have focused on innovation and/or entrepreneurship. Because business and industry is in a transitional phase, it is very important to make NHH students better equipped to create new enterprises or help others make a new enterprise grow. The aim of the Lehmkuhl grant is, in other words, to support NHH's focus on innovation and entrepreneurship.
The grounds for awarding the grant emphasise that the master’s thesis demonstrates how advanced statistical methods can be used to help owners of big dry cargo ships to plan which routes they should run.
The shipping market is highly complex and uncertain, and poor decisions can have major negative consequences. Great insight and many years of experience are therefore needed to plan the running of a fleet. The paper presents an automated system, which, based on the processing of large quantities of data for a host of variables, suggests which routes the different ships should be assigned to.
The system is based on methods for machine learning, which means that it can improve itself based on previous actions. The methodology was tested on real data from the period 2017–2020. The results show that you can achieve a significant increase in profits compared to the expected return from different stakeholders in this market.