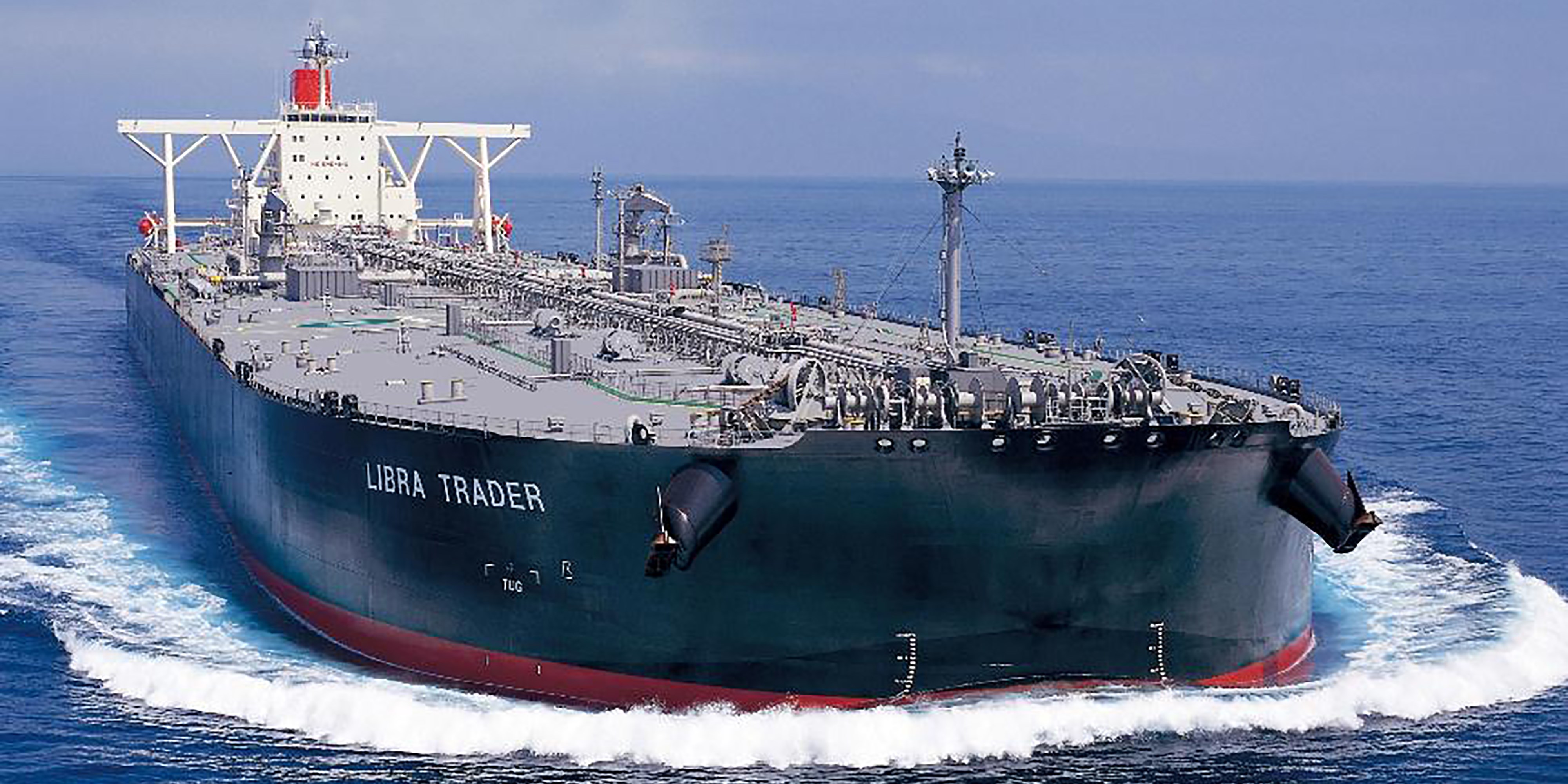
NHH students make machine learning algorithm breakthrough, beating the cape markets by 10%
Just how much machine learning algorithms can deliver to capesize earnings has been quantified in a landmark new piece of research, by students supervised by Roar Ådland.
For their master thesis two NHH students, Herman Bomholt and Torsten Thune, deployed machine learning algorithms to simulate the cape markets over the 2017 to 2019 period with the results proving to be about 10% or $1,700 higher per day per vessel than the benchmark Baltic time charter average.
The study captured about two thirds of the theoretical maximum potential from optimising the Asia – Australia/Brazil trip sequencing. Importantly, the research did not make use of either AIS or fixture data. It did include various commodity, foreign exchange and other macro data.
Their research was featured, on 19 June 2020, at Splash247.com, who followed up with an interview with the two students in their next issue, on 24 June:
The students who beat the cape markets
When Splash carried news on Friday that a pair of Norwegian students had developed machine learning algorithms that had beaten the cape markets, the story spread like wildfire, read by more than 100,000 people and counting. Here was quantifiable evidence that machines can replicate - and improve upon - human behaviour in an area of shipping that has always been so centred around human judgement and the industry’s famous love for gut feeling decisions.
For their master thesis two students at the Norwegian School of Economics (NSE), Herman Bomholt (pictured right) and Torsten Thune, deployed algorithms to simulate the cape markets over the 2017 to 2019 period with the results proving to be about 10% or $1,700 higher per day per vessel than the benchmark Baltic time charter average.
The NSE’s shipping department, led by Dr Roar Adland, has an ongoing research project focusing on smart digital contracts and commercial management, part of which deals with data-driven analytics for chartering. This thesis builds on an earlier published paper penned by Adland, Stein Wallace and Vit Prochazka which looked at the theoretical potential for optimising chartering in the sense of where a ship ought to be sent next. The new study has implemented this optimisation in a real-life data-driven framework using modern machine learning methodology.
Thune and Bomholt’s study captured about two thirds of the theoretical maximum potential from optimising the Asia – Australia/Brazil trip sequencing. Importantly, the research did not make use of either AIS or fixture data. It did include various commodity, foreign exchange and other macro data.
Full details of the study are to be released later this year and the thesis has yet to be peer reviewed so the students are not giving away too much details yet, but they were still happy to chat with Splash Extra.
The big news is that the framework developed by the students can be transferred to other ship types.
“As of now, the input data used, and the models themselves, are tailored to support capesize market analysis. However, this can easily be adapted to other ships by including or substituting data from specific market drivers relevant to the vessel of choice,” says Bomholt. Bomholt explains the decision not to use AIS data as input data was because of the limited availability and quality prior to 2014. This would have decreased the time horizon significantly, reducing the chartering period used for evaluation.
“We believe AIS data could be an important addition to such models for future work, as quality and availability increases,” Bomholt says.
While there are a host of chartering platforms coming to the market very few have made public how they can consistently beat the markets. What Bomholt and Thune have done is to put a percentage figure on how their data-driven development can beat the Baltic. Harnessing data to make profits in shipping has been something of a Holy Grail for shipping. Ought brokers to be worried by the students’ breakthrough research? Not necessarily, says a modest Thune - the human touch in chartering is not going away any time soon, he insists.
“Naturally, machine learning today cannot directly replace intuition and deep experience in the field,” Thune says, adding: “Still, we believe it could serve as a decision support system in operational planning by extracting signals and relationships useful when designing chartering strategies.”
For more on the future of chartering check out our special correspondent’s take on the matter on this month’s Opinion page.